2 Machine Learning Models Found to Predict Hospitalization in SLE
1 best for short-term, the other for longer assessments: study
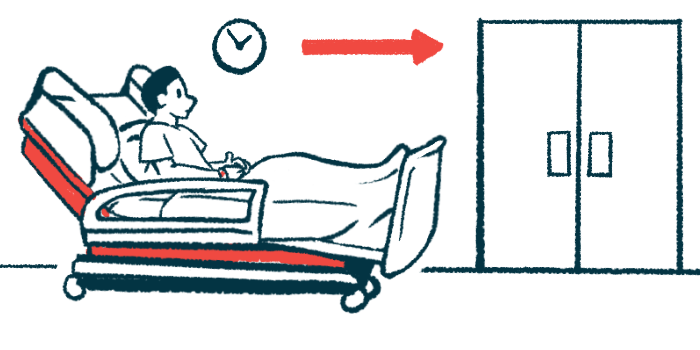
Using clinical data collected over time, two machine learning computer models were found to accurately predict inpatient hospitalization due to systemic lupus erythematosus (SLE) — the most common form of lupus.
One method was most accurate in using short-term clinical assessment data to foretell future hospitalizations, while the other worked best for more extended assessment periods.
“Our experimental results demonstrate that both methods can be effective for our task,” the researchers wrote of the machine learning models, adding that “each has its strengths and limitations.”
The findings were reported in a study, “Comparing two machine learning approaches in predicting lupus hospitalization using longitudinal data,” published in the journal Nature Scientific Reports.
SLE is a chronic autoimmune disease that affects multiple systems in the body, with symptoms that can vary from patient to patient and fluctuate over time. In some cases, people with the condition can experience severe disease flare-ups, requiring inpatient hospital care.
Predicting future outcomes
Predicting disease outcomes in chronic medical conditions like SLE is challenging, but necessary to support patient monitoring and ensure appropriate treatment.
Computer models have been used to predict SLE outcomes using demographic information together with clinical data collected over differing time periods. Such information is called longitudinal data.
However, current models are limited in their ability to accurately process data over time.
Now, researchers at Fordham University, in New York, and Harvard Medical School, in Massachusetts, tested two new machine learning approaches using time-based clinical data to predict future hospitalizations in people with SLE.
The team collected multicenter longitudinal patient data from the electronic health records of 925 SLE patients enrolled in the Massachusetts General Brigham (MGB) lupus study. Data from each patient included five demographic features, as well as 52 clinical features collected at six-month intervals. Such features were clinical characteristics, SLE medications, laboratory test values, and healthcare utilization, among others.
Models incorporated clinical data at six, 12, 18, and 24 months (two years) to accurately predict SLE hospitalization at three, six, nine, and 12 months (one year) in the future.
The first computer prediction approach, called Differential, accounted for time-based dependencies in clinical data collected longitudinally by introducing so-called lagged variables to capture disease progression. The second method, known as long short-term memory or LSTM, is a state-of-the-art deep learning model designed to analyze time-dependent data.
Analyses revealed the Differential approach’s overall performance decreased as the patient monitoring period increased for all future time-point predictions. The LSTM model showed the same pattern with three and six months of patient monitoring, but reversed with later time points.
The Differential approach was most effective at predicting future SLE hospitalization with short (six- and 12-month) observational intervals, but it lost efficacy as observation time increased. The prediction accuracy for less than 18 months of observation was 88%, which dropped to about 50% with 36 months (three years) of observation.
In contrast, the LSTM model was more effective at predicting future SLE hospitalization with more extended observational periods. LSTM’s predictive accuracy was 74% with 24 months of observation, while the Differential approach’s had a predictive accuracy of 64%.
Regarding the predicted time to hospitalization, or prediction horizon, LSTM’s best overall performance improved from 53% to 81% across different prediction horizon values. In contrast, the Differential method maintained a stable performance across all prediction horizon values. Also, LSTM outperformed Differential when the prediction horizon was longer than six months.
These data suggest the LSTM model should be used when observational periods are longer than 18 months and the prediction horizon is longer than six months. The Differential model is recommended for use in all other cases, the researchers noted.
The Differential approach reached maximum accuracy for a six-month assessment period and a 12-month outcome period. The LSTM model achieved maximum accuracy for 24 months of clinical feature assessment and nine months of outcome assessment.
Lastly, the team found that for 24 months of observation, the LSTM model achieved the highest accuracy in predicting SLE hospitalization nine and 12 months in the future. With a predictive horizon of three and six months, the Differential method was more accurate, but accuracy decreased with increasing observation time.
“These models can be applied accordingly to predict future SLE hospitalizations from various patient monitoring periods ranging from 6 [months] to 36 [months],” the researchers wrote. “We further suggest 6 [months] and 24 [months] as the desirable observation windows for short- and long-term assessment horizons, respectively.”
“Our approach could be applied to other clinical conditions to leverage time-dependent [electronic health records] data to predict longitudinal health outcomes,” they concluded.