Machine Learning, After Fine-tuning, May Be Useful for SLE Classification, Study Suggests
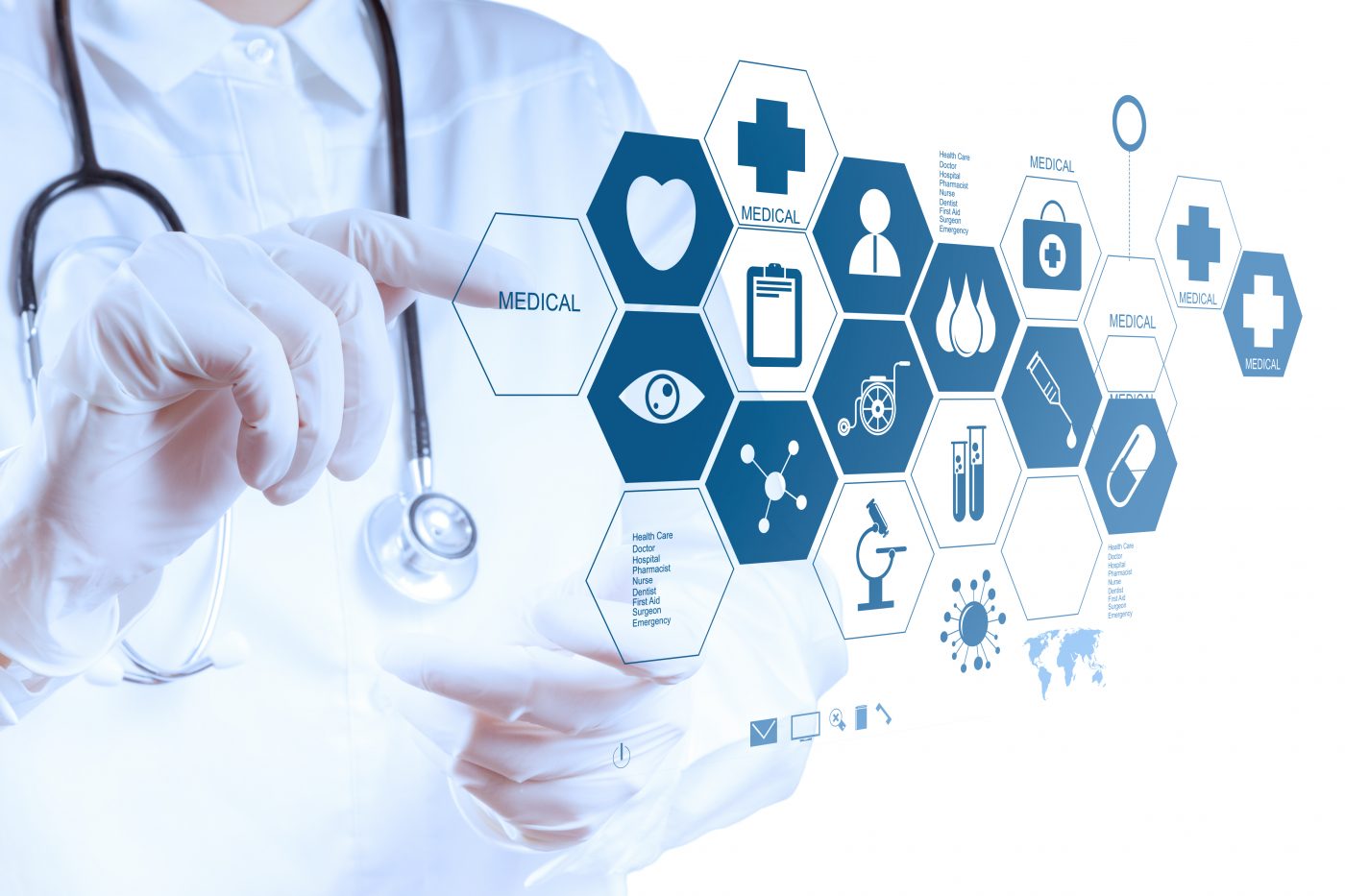
Machine learning analysis strategies can help predict disease status in people with systemic lupus erythematosus (SLE), a study shows. Still, technical variability inherent to each clinical analysis method can represent a roadblock in this process.
Fine-tuning of machine learning algorithms and parameter sets may help reduced technical “noise” caused by such variability, the researchers suggest. They said this would generate “sufficient accuracy to be informative as a standalone estimate of disease activity.”
Titled “Machine learning approaches to predict lupus disease activity from gene expression data,” the study was published in Nature Scientific Reports.Â
Diagnosing and classifying SLE is a challenge for clinicians because the disease is so varied in how it presents. One proposed way to classify this type of lupus is based on gene expression levels, which evaluate which genes are “turned on” and by how much.
Several attempts have now been made to classify SLE patients based on gene expression data. However, none was successful in identifying a reliable marker that could allow an individual with the disease to get useful information from such a test — which is the ultimate goal.
To more thoroughly assess this question, researchers from the RILITE Foundation and AMPEL BioSolutions, with collaborators from George Washington University, used machine learning approaches to evaluate three different data sets collected from SLE patients, available from previous studies.
The investigators employed several machine learning techniques to analyze the information, or learning data set, and build computer algorithms that could classify it. For example, one algorithm created would find certain genes associated with active disease status and then use those genes as predictors in the analysis of new samples.
Next, the team tested those “learned” algorithms on the rest of the data, or training data set. The aim was to evaluate how well the algorithms could predict disease status based on gene expression data.
However, a problem quickly became apparent. When the computers “learned” using one of the three data sets as the training data set, and the remaining two as the testing data set, the results were pretty much useless. Basically, there was just too much technical variation between the different data sets for them to be comparable.
“Gene expression values have little to no utility when attempting to classify unfamiliar samples,” the researchers said. “When the training and test data come from different data sets, the classifiers learn patterns that are unhelpful for classifying test samples.”
Some strategies, like focusing on particular groups of genes associated with specific types of cells, improved the results. Using data from all sets to form both the testing and training data sets also proved to be helpful. This suggested that it was the technical reliability of the data itself — not the machine learning algorithms — that was the main obstacle in constructing a predictive model.
“If such a test [of gene expression] could be reliably free from technical noise, it is likely that raw gene expression would perform very well,” the investigators said.
The study used for gene expression data was retrieved from microarrays — laboratory tools used to detect the expression of thousands of genes at the same time — that use molecular probes to assess the gene’s levels. Variations in these probes, between different commercially available platforms, are likely culpable in the detected variations.
Researchers speculated that either standardizing these platforms, or using other techniques, like RNA sequencing (or RNA-seq), might help fix this problem. In RNA-seq, all the genes being made by the cells in the sample are sequenced, and the number of sequences of a given gene that are detected can provide information about gene expression.
The investigators also believe that implementing such tests to be analyzed by machine learning strategies has substantial promise.
“Integration of our approaches with emerging high-throughput patient sampling technologies could unlock the potential to develop a simple blood test to predict SLE disease activity,” the researchers said.
“Our approaches could also be generalized to predict other SLE manifestations, such as organ involvement,” they added.