False Diagnoses in Big Databases a Major Problem for Research, Study Says
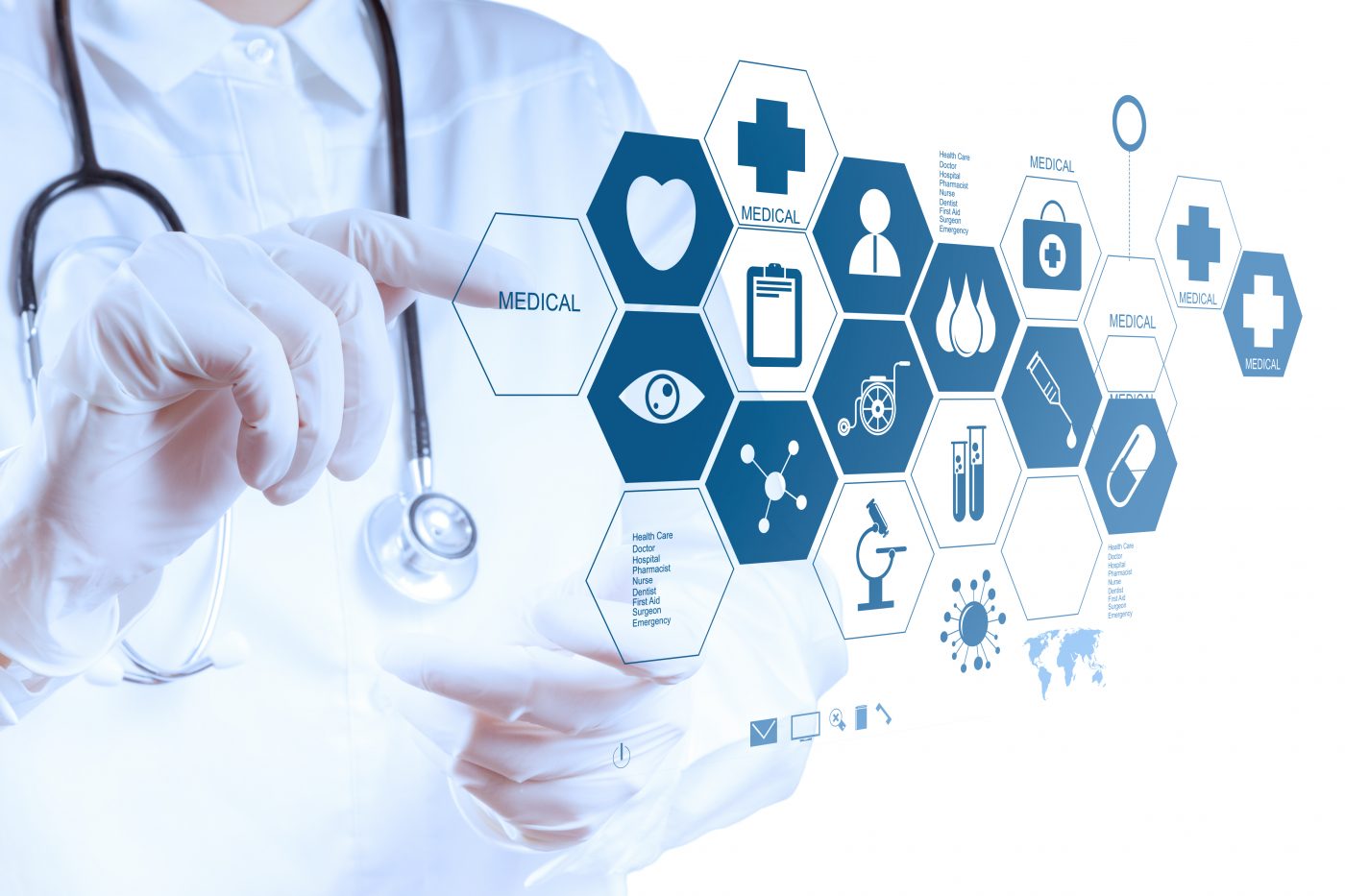
In conditions with complicated diagnostic processes, like systemic lupus erythematosus (SLE), research done using healthcare databases may be affected by tentative diagnoses, a study reports.
Using an Estonian database, researchers found that only 60% of people with a diagnostic code for SLE actually had the condition, as confirmed by their healthcare providers.
The study, “Administrative database as a source for assessment of systemic lupus erythematosus prevalence: Estonian experience,” was published in the journal BMC Rheumatology.
Many epidemiology studies rely on data that is collected for healthcare administration, such as insurance billing. This relatively new method can be advantageous because it provides data on many people all at once, allowing for studies with large populations.
However, these databases aren’t designed for research. As such, they may have under-appreciated drawbacks — one of which is the possibility of false-positive diagnoses.
In this study, researchers looked for false-positive diagnoses of SLE in the Estonian Health Insurance Fund (EHIF) database over a four-year period beginning in 2006. The EHIF insures more than 95% of the 1.3 million people who live in Estonia. In the database, SLE has the code M32.
The researchers retrieved data for 9,342 billing episodes from 2006 to 2010 marked with M32 that were applied for 677 people. The researchers divided these patients into two groups based on how many times they had been given this label by a certified rheumatologist. A rheumatologist is a doctor who specializes in immunology; there are only 20 in the country of Estonia.
A total 326 people had been assigned M32 by a rheumatologist four times or more. Researchers randomly selected 20% of these patients for further review, contacting their doctors to get more data and determine whether the SLE diagnosis was correct. In cases where there was an error, the doctors were asked to describe why the error had been made.
For the 351 people who had been marked M32 by a rheumatologist less than four times, the researchers used the same review process. However, the entire group of patients were reviewed, not just one-fifth. The researchers reasoned that multiple diagnoses by a rheumatologist would make a false diagnosis less likely; four such determinations was chosen as the cut-off value.
Among the assessed cases in the first group, all but one were confirmed as having an SLE diagnosis. (The misdiagnosis was a complicated case of secondary syphilis, the researchers noted.)
In the second group, however, only 79 patients — 23% of the cases — were confirmed as having SLE. Researchers said 31 people (9% of patients) didn’t have available data. The remaining 241 people — 68% of the initial diagnoses — were found to have other medical issues, mostly immunological conditions.
The most common reasons for misdiagnoses among general practitioners were referrals intended to actually get the patient to a rheumatologist for a proper diagnosis — which accounted for 39.5% of errors — and coding errors, which accounted for 32.1% of misdiagnoses. Similarly, among rheumatologists, nearly three-quarters of the false-positives were referrals for future testing to confirm a diagnosis.
The high number of coding errors, the researchers said, “could presumably be attributed to the beginning of the study period when prescriptions were still handwritten.” The investigators said the code “M32” might have been mistaken for other similar codes, such as H32 or N32, because of bad doctor handwriting. An electronic system was not implemented in Estonia until 2010. As such, the researchers propose that these errors would be less common in the future.
The researchers said this study illustrates the importance of understanding what data is or isn’t in a database, and how reliable that data is, when designing a scientific study. Although the researchers don’t think their false-positive rates are necessarily applicable in other systems — which probably have numerous differences — the overarching concept is that scientists need to be cognizant of the validity, and the potential drawbacks, of using big databases.