Novel Biomarkers May Help Predict Response to Lupus Nephritis Therapy
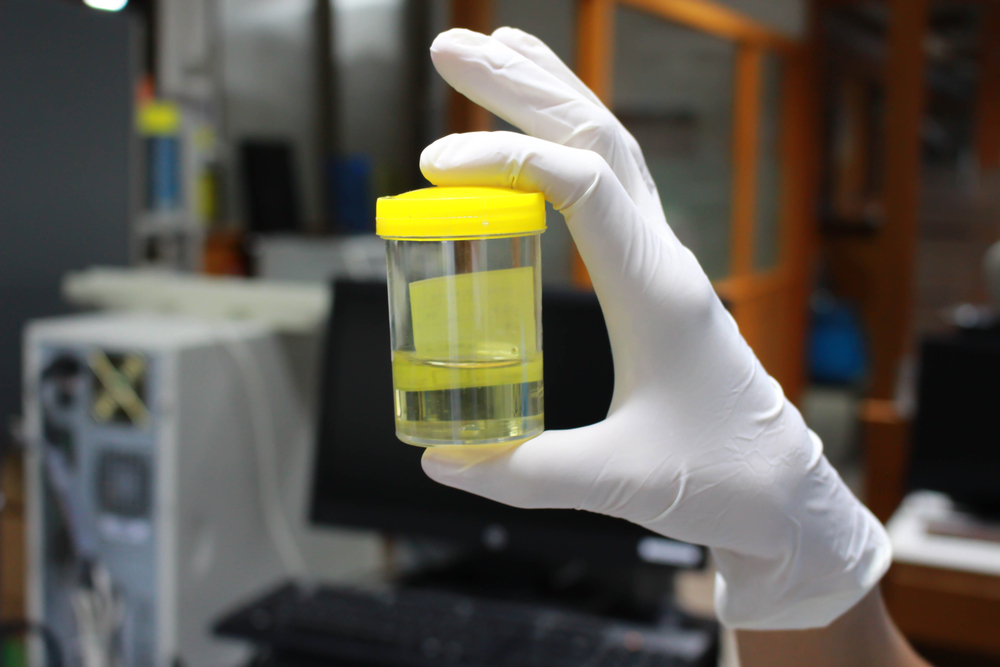
Researchers have identified novel biomarkers for lupus nephritis (LN), a disease that affects several patients with systemic lupus erythematosus (SLE), according to a study published in the journal Arthritis & Rheumatology. The authors reported these biomarkers can accurately indicate disease development and predict therapeutic effectiveness.
The study is titled “Development of Biomarker Models to Predict Outcomes in Lupus Nephritis” and was conducted by researchers from the Medical University of South Carolina.
LN leads to kidney failure in up to 50% of patients within five years. The American College of Rheumatology guidelines for the treatment of LN recommend that induction therapy (first treatment given to a patient) must be changed when response to therapy has not occurred within 6 months. However, response to therapy is difficult to define in LN patients, and renal damage can occur while waiting for this result.
The assessment of traditional biomarkers to evaluate LN response is performed through blood pressure measurements, urinary sediment, urinary protein-to-creatinine ratios, and surrogates of renal function, among others. But these markers have low sensitivity and vary among LN patients. Also, even when clinicians use machine learning models to evaluate these markers, they show only 69% accuracy in predicting a LN diagnosis.
For these reasons, it is crucial to find a tool to better define response to therapy and guide clinicians when starting patients’ treatments.
“We saw our colleagues’ frustration in trying to come up with predictive models,” said the senior author of the article, Jim Oates, MD, in a news release. “The traditional markers we use in clinic today have quite limited predictive capacity. All lupus patients have varying degrees of kidney damage and levels of involvement of the different kidney structures. So, we wanted to account for this heterogeneity and the stages of disease progression. We wanted to include markers for pathways of inflammation as well as for damage,” said Oates.
To do so, researchers analyzed urine samples from 140 LN patients who had not started induction therapy, and looked for novel biomarkers that could be used in combination with machine learning models to help predict outcomes better than standard biomarkers.
Researchers found that markers most predictive of response were markers of inflammatory cell activation (cytokines), signals for homing to the kidney (chemokines), activation of inflammatory cells (growth factors), and damage to resident cells.
The results supported the idea that the use of these biomarkers with machine learning techniques may provide a reliable tool to predict disease outcome.
“This could apply to any model where there is kidney inflammation leading to damage,” said Oates. “It’s proof of concept for other kidney diseases that you can take a discovery model and incorporate machine learning to develop and validate predictive models.”
The next step will be to validate these biomarkers in a larger patient population. According to Oates, their model for outcome prediction can be translatable to the clinic setting faster and less expensively than a FDA validation process, and may take into account several factors that affect outcomes, such as the economic and societal status of the patients.